Normalisation Annotation in CHF
Annotation scheme
Each entity mention annotated during the entity mention annotation effort is associated with a unique concept listed in the UMLS Metathesaurus. This is a large terminological resource that contains entries for millions of biomedical and health related concepts. Each distinct concept in the UMLS Metathesaurus is assigned a unique identifier code called a Concept Unique Identifier (CUI).
The normalisation annotation in PhenoCHF involves a CUI being assigned by a medical doctor to all instances of the the following types of entity mentions (please see the Entity Mention annotation page for details of each of these entity types:
- Cause
- Risk Factor
- Non-tradtional Risk Factor
- Signs & Symptoms
The normalisation annotation in the PhenoCHF corpus provides the means to develop systems that can perform normalisation automatically. For reasons such as those introduced below, this can help to considerably increase the utilty of methods that recognise entitites and relations.
Motivation
A given concept can be expressed in text in many different ways. Resources such as the UMLS Metathesaurus usually list some synonyms for each concept, i.e., different ways in which the concept could be expressed in text. However, there tend to be many more ways of mentioning a concept in text than those that correspond to the synonyms listed for the concept in terminological resources. Part of the problem is that such resources are usually manually curated. This means that is impossible to keep track of all possible ways in which a concept could be mentioned in text, especially according to the highly creative nature of language. Accordingly, when an entity mention is recognised in text, it can be difficult to determine the exact concept to which it refers.
Linking entity mentions to the concepts that they describe (in a process called normalisation) can be important for a number of reasons. For example:
- When using a search system to find all documents that mention a concept, a user is likely to use one or two well-known synonyms for the concept as their search terms. However, it would be impossible for the user to enumerate every single way in which the concept could be mentioned in text. Accordingly, many documents that are potentially relevant to the user's query may be overlooked. As such, systems that can automatically detect all documents in which a concept is mentioned, regardless of how it is mentioned, can be very useful in helping to find more documents that are relevant to the user.
- It can be important to gather different types of information that are specified about given concept (e.g., all risk factors of a given disease), and which are possibly dispersed amongst different documents. The relation annotation in PhenoCHF provides the means to develop systems that can automatically find associations between entity mentions. If it is possible for each of the entity mentions that are involved in a relation to be linked to the unique concept that it represents, then the extracted information can become far more useful. For example, all types of relations that involve a given concept in a huge document collection can be retrieved, regardless of how that concept is expressed in text. This can be particularly important, according to the fact that that different (and often complementary) types of information are often reported in different textual sources (e.g., narrative EHR records and literature articles), given that each has a different focus.
Concept variation
Typically, synonyms of concepts listed in terminological resources tend to represent "standard" ways of referring to the concept (typically noun phrases), which often do not reflect how the concept is actually mentioned in text. Variation amongst mentions of concepts is particularly apparent in narrative EHR reports. For example, concepts may be mentioned in text as simple noun phrases (e.g. progressive renal failure), noun phrases followed by prepositional phrases (e.g., increasing dyspnea on exertion) and complete clauses or sentences (e.g., jugular venous pressure is elevated).
Table 1 illustrates some of the different types of variation that can occur amongst mentions of the same concept.
Type of variability | EHR mentions | Article mentions |
---|---|---|
Synonymy | Sodium overload Drop in blood pressure | Hypernatremia Hypotension |
Syntactic structure | Left ventricular is dilated Mild mitral calcification | Left ventricular dilatation Calcification of mitral valve |
Word ordering | Cardiac output decreased | Decreased cardiac output |
Morphological variation | Hyperkalemic | Hyperkalemia |
Concept distribution in narrative EHR reports and articles
The gold standard normalisation annotations in PhenoCHF reveal that 835 concepts are mentioned in the corpus as a whole. Of these, 184 occur in both narrative EHRs and literature articles. This shows that while a significant number of concepts are mentioned both types of text, there are many concepts that are only mentioned in one of the two text types. This provides strong evidence that different types of information are specified about these common concepts in the different text types, thus demostrating the need to link together mentions of concepts that occur across different text types.
Figure 1 illustrates the distribution of the concepts that overlap between the two text types. Although in narrative EHRs, the dominant concept type is Signs & Symptoms, there is a far greater emphasis in literature articles on discussing Causes. However, the frequent mention of possible causes of observed signs and symptoms in narrative EHRs, and the observation that literature articles will often summarise these potential causes, helps to explain why Cause is the phenotype entity type with the greatest overlap between EHRs and literature articles.
Figure 1. Distribution of types of concepts that overlap between narrative EHRs and lierature articles
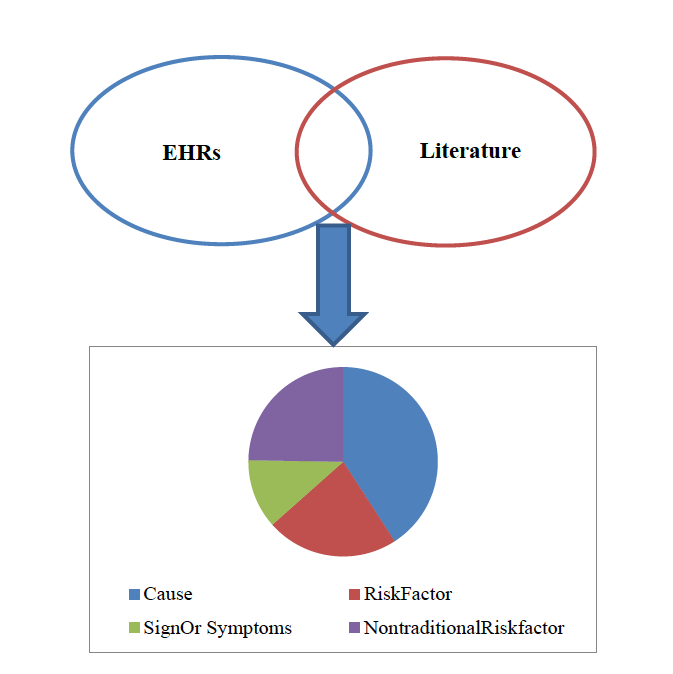
Featured News
- 1st Workshop on Misinformation Detection in the Era of LLMs - Presentation slides now available
- Prof. Ananiadou appointed Deputy Director of the Christabel Pankhurst Institute
- ELLIS Workshop on Misinformation Detection - Presentation slides now available
- Prof. Sophia Ananiadou accepted as an ELLIS fellow
- BioNLP 2025 and Shared Tasks accepted for co-location at ACL 2025
- Prof. Junichi Tsujii honoured as Person of Cultural Merit in Japan
Other News & Events
- AI for Research: How Can AI Disrupt the Research Process?
- CL4Health @ NAACL 2025 - Extended submission deadline - 04/02/2025
- Invited talk at the 15th Marbach Castle Drug-Drug Interaction Workshop
- Participation in panel at Cyber Greece 2024 Conference, Athens
- Shared Task on Financial Misinformation Detection at FinNLP-FNP-LLMFinLegal