Entity Mention Annotation in CHF
Annotation scheme
Our annotation scheme for entity mentions aims to identify words and phrases that describe a number of types of concepts that are highly relevant to phenotype phenomema. Table 1 defines the types of concepts whose mentions are annotated on in the PhenoCHF corpus.
Concept Type | Description | Examples of mentions |
---|---|---|
Cause | Any medical problem that contributes to the occurrence of CHF | chronic renal insufficiency, hypertension |
Risk Factor | A condition that increases the chance of a patient having the CHF disease | obesity, type 2 diabetes, high cholesterol |
Sign & Symptom | Any observable manifestation of a disease which is experienced by a patient and reported to the physician | productive cough, nausea, vomiting |
Non-traditional risk factor | Conditions associated with abnormalities in kidney functions that put the patient at higher risk of developing signs ∓ symptoms and causes of CHF | iron deficiency, anemia |
Organ | Any body part | lungs, abdomen |
Chief Complaint | Mentions of CHF | CHF, congestive heart failure |
Entity mention statistics
All mentions of the concepts of the types shown in Table 1 were annotated in each document of PhenoCHF. The total counts of each type of entity annotated in each part of the corpus (i.e., narrative EHR reports and literature articles) are shown in Table 2
Concept Type | No of annotated mentions in narrative EHR reports | No of annotated mentions in literature articles |
---|---|---|
Cause | 1320 | 1107 |
Risk Factor | 1335 | 408 |
Sign & Symptom | 2449 | 304 |
Non-traditional risk factor | 308 | 329 |
Organ | 432 | - |
Distribution of entity mentions in PhenoCHF
Figure 1 provides on overview of the distribution of the mentions of phenotype-related concepts in PhenoCHF. In discharge summaries, there is large emphasis on describing the signs and symptoms of the disease, but these play a much less significant role in scientific articles, where the dominant topics are non-traditional risk factors and the etiology of CHF.
Figure 1. Distribution of entity mention annotations in PhenoCHF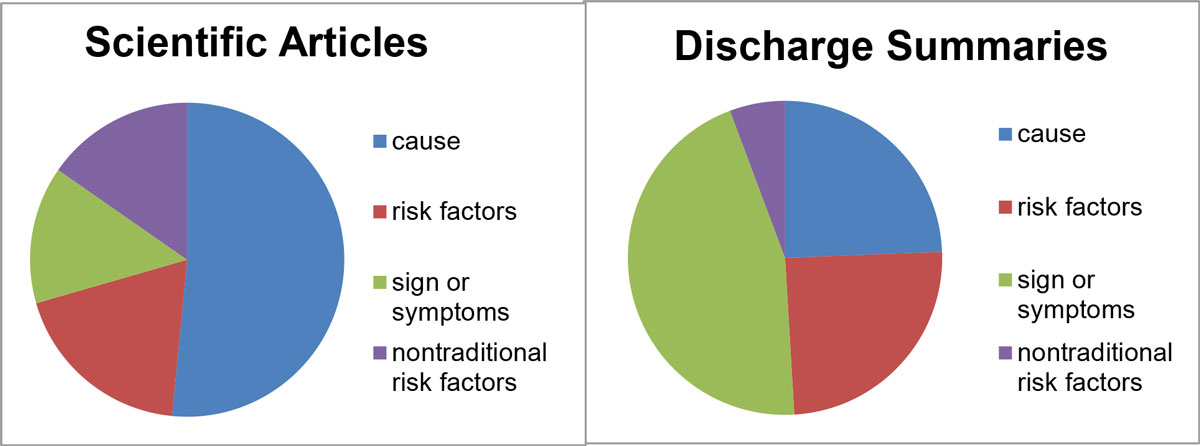
Agreement
The entity annotations were undertaken by two medical doctors. The quality and consistency of the annotations were verified through the calculation of inter-annotator agreement (IAA). We calculated IAA in terms of F-Score, and found that high levels of agreement were acheived. We calcluated both exact span matches, where the start and end of the annotated text spans chosen by both annotators must match exactly, and relaxed span matches, where it is sufficient for the annotated text spans to include some common parts. The IAA statistics, in terms of F-score, are shown in Table 3.
Agreement Type | Narrative EHRs | Literature articles |
---|---|---|
Exact Match | 0.82 | 0.69 |
Relaxed Match | 0.92 | 0.77 |
Featured News
- AI for Research: How Can AI Disrupt the Research Process?
- ELLIS Workshop on Misinformation Detection - Presentation slides now available
- 1st Workshop on Misinformation Detection in the Era of LLMs (MisD)- 23rd June 2025
- Prof. Sophia Ananiadou accepted as an ELLIS fellow
- Invited talk at the 15th Marbach Castle Drug-Drug Interaction Workshop
- BioNLP 2025 and Shared Tasks accepted for co-location at ACL 2025
- Prof. Junichi Tsujii honoured as Person of Cultural Merit in Japan
- Participation in panel at Cyber Greece 2024 Conference, Athens
- New Named Entity Corpus for Occupational Substance Exposure Assessment
Other News & Events
- CL4Health @ NAACL 2025 - Extended submission deadline - 04/02/2025
- Shared Task on Financial Misinformation Detection at FinNLP-FNP-LLMFinLegal
- FinNLP-FNP-LLMFinLegal @ COLING-2025 - Call for papers
- Keynote talk at Manchester Law and Technology Conference
- Keynote talk at ACM Summer School on Data Science, Athens